▤ 목차
Describing probability distributions and probability distributions with multiple variables
Describing Distributions
Skewness and Kurtosis: Moments of a Distribution
When we multiply the random variable X with the power of an integer n, we call it the nth moment.
Skewness and Kurtosis - Skewness
Without calculating, intuitively compare the expected value and the variance of two games. Which of the following statements is true? Select all that apply.
- The expected value of game 1 is greater than game 2.
- The expected value of game 1 is equal to the expected value of game 2.
- The variance of game 2 is greater than game 1
- The variance of game 1 is equal to the variance of game 2
Answer
2, 4
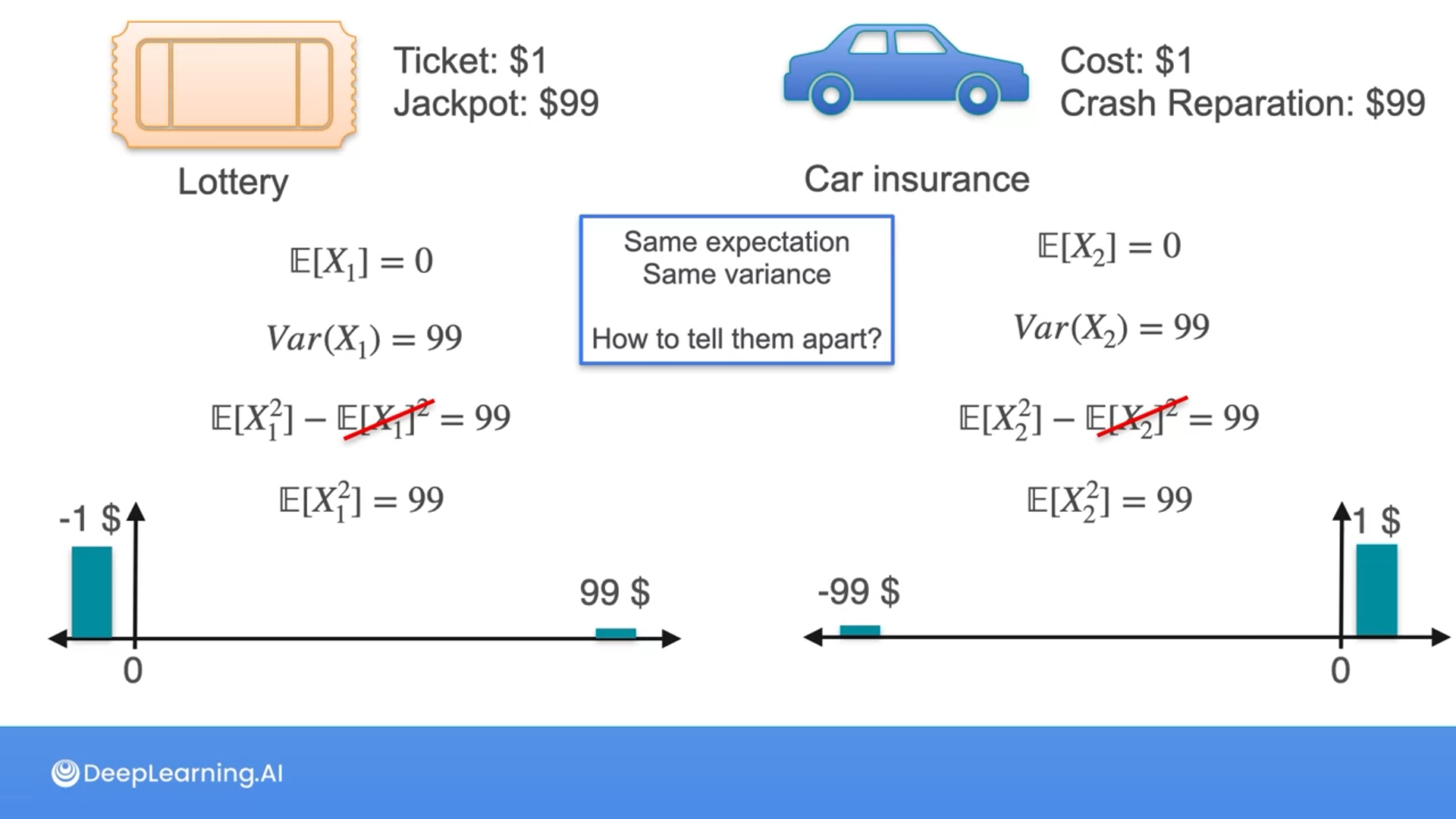
With the given example, we have equal expected values and standard deviations, but they are different, so we need other methods to compare them.
So we use the third moment (apply cube to X), which gives us whether the distribution is skewed to a certain direction or not.
Then we apply standardization to get the skewness.
Skewness and Kurtosis - Kurtosis
Another method is required to compare distributions when the expected value, variance, and skewness are the same between them.
Kurtosis is the standardized fourth moment.
Kurtosis tells us how thick the tails (endpoints) of the distribution are.
Thick tails refer to more extreme values (outliers) and sharper central parts (less data in the center).
Thin tails refer to less extreme values and smoother central parts (more data in the center).
All the information provided is based on the Probability & Statistics for Machine Learning & Data Science | Coursera from DeepLearning.AI